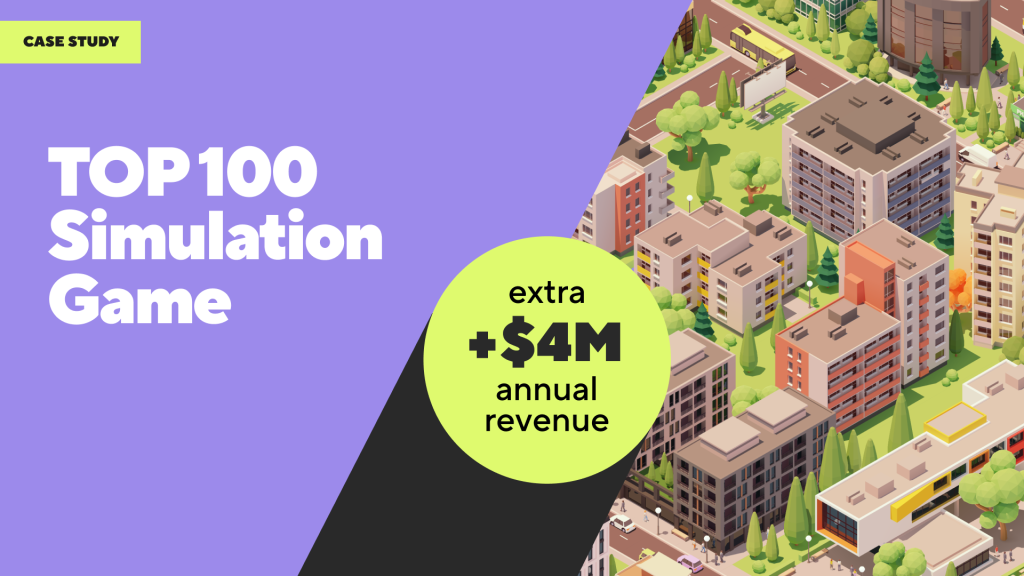
You’ve created a game players love, but revenue isn’t growing. It’s a common scenario. Luckily, at SuperScale we’re experts in identifying specific IAP monetization areas that can help developers unlock the revenue their game deserves.
In this instance, our client felt their top 100 grossing game wasn’t earning the revenue it should be. So we got to work.
What our performance evaluation identified
- Lower conversion in certain player segments, like veteran gamers focused on late-game currencies and casual players focused on fashion.
- Some consumables, including late-game currencies, were hard to get without dedicating crazy amounts of game time, so a lot of players had stopped pursuing them.
- Excessive offer discounts were cannibalizing monetization and driving currency hoarding.
We decided to run an in-game offer personalization strategy to connect with and convert players without the need for deep discounts.
In 3 months we aimed to
- Create new pricing and content modeling
- Connect these to A/B test structure
- Set up monitoring and reporting
- Run the pilot A/B test
Our goal
Revitalize year-on-year revenue for this leading mobile game.
Harnessing probabilistic and machine learning to generate over 50,000 unique daily offers
We set to work building a personalization engine that generated more than 50,000 unique offers each day. The engine would learn players’ behavior and spending patterns, and configure game assets into unique offers for each player.
Players would receive up to 5 unique offers each day, 3 of which they’d see instantly, with a further 2 queued and waiting. Each day offers would change based on the player’s behavior, creating a fresh, customized shopping experience that ensured gamers kept coming back for more.
Throughout the process, we used A/B to evaluate the impact of our improvements, tweaking as we went. Initially we tested 10% of players, broadening our testing field to 30% and later 90%.
We developed the initial models, with the necessary data pipeline and transformation, within a month and a half. The success of the first A/B test meant we could keep up the relative performance numbers while scaling absolute revenue significantly.
We’re all about probabilistic and machine learning models (XGBoost, Neural Networks) to understand not only the price, but also the content of the offers.
The results after in-game special offer personalization
The new personalization boosted game revenue by 11%. The benefits extended to UA, unlocking an extra 23% in spend with no fall in retention.
Other results included:
+7.25% daily paying users
+4% conversion
Want to know how we can drive your game’s success? Get in touch today.