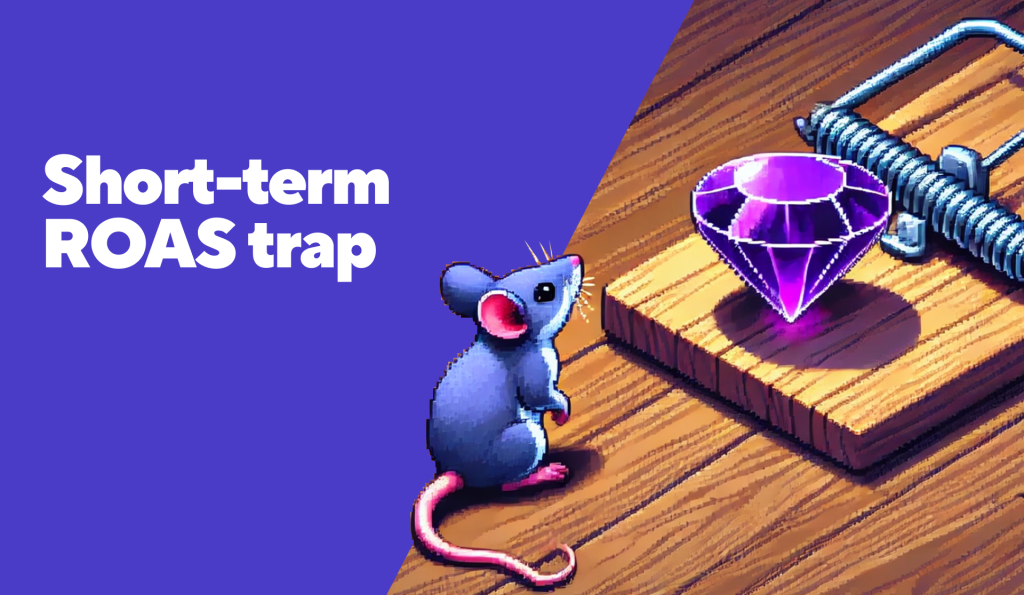
In mobile gaming, User Acquisition (UA) is the engine that drives growth. Yet, scaling UA campaigns effectively is more challenging than ever. Many UA managers still rely on short-term metrics like Day 7 or Day 14 return on ad spend (ROAS) to gauge success. While these figures provide a quick snapshot of performance, they offer a limited perspective, often leading to misallocated budgets and overlooked opportunities for sustainable growth.
The problem: Short-term metrics and outdated benchmarks
A major challenge UA teams face is making critical budget and strategy decisions based on outdated benchmarks. Traditional ROAS targets are often static, failing to account for the evolving nature of monetization and player behavior. This rigidity leads to decision-making that is reactive rather than proactive.
Day 7 or Day 14 ROAS might indicate initial campaign performance, but they do not reveal the full profitability over the user’s lifecycle. As a result, UA teams relying solely on these short-term metrics risk misallocating budgets, missing signals of long-term revenue potential, and reinforcing ineffective strategies.
Adding to this complexity, the accuracy of predictions depends on various factors. Without understanding the confidence interval of these predictions, UA managers must rely on intuition to gauge risk, increasing uncertainty in budget allocation.
The consequences: Avoidable underperformance
Overreliance on short-term ROAS targets results in several key issues:
- Inefficient budget allocation: Campaigns that could generate strong long-term value may be cut off too early, while short-term winners may be overfunded, leading to suboptimal results.
- Missed opportunities to scale: UA teams may not recognize improved long-term monetization trends if they rely only on static benchmarks. Without adaptive ROAS predictions, they underspend and leave potential revenue untapped.
- Delayed strategic adjustments: Short-term ROAS targets do not capture real-time trends. Without predictive models that evolve with cohort data, UA teams react too late to optimize campaigns.
- Lack of visibility into LTV shifts: As player behaviors change, outdated benchmarks fail to account for shifts in Lifetime Value (LTV). Without predictive models that update dynamically, UA teams cannot adjust their strategies in time to capture emerging opportunities.
The solution: Adaptive ROAS predictions
In a competitive industry with thin margins, relying on incomplete and outdated data can stifle growth. The problem worsens when UA teams and product teams operate in silos, as game design decisions influence LTV patterns and, consequently, UA strategies.
To overcome these challenges, UA teams need predictive models that:
- Adapt to evolving cohort behaviors rather than relying on static benchmarks.
- Provide insights across different granularities—platforms, UA channels, and creatives—so that budget allocation aligns with long-term profitability.
- Incorporate confidence intervals, enabling UA managers to quantify risk rather than relying on intuition.
- Account for SKAdNetwork (SKAN) limitations on iOS and enable more precise forecasting despite restricted data availability.
Understanding and improving the accuracy of ROAS predictions is crucial for making informed, data-driven decisions. Predictive models must be evaluated for precision, ensuring that UA teams can trust the insights they generate.
Curious about how to leverage adaptive ROAS predictions? We have the solution. SuperPlatform takes the guesswork out of UA management . In our next article, we’ll explore how SuperPlatform drives UA success. Want to learn more about SuperPlatform? Read here.